Executive Summary
In an increasingly competitive global market, effective pricing strategies and quick decision-making are essential for sustaining and growing market share. This white paper explores how the Tversky algorithm was leveraged to develop a sophisticated pricing tool for a multi-billion-dollar US conglomerate that recently acquired a new brand of LED products. By integrating advanced AI and machine learning, the tool provided real-time, data-driven pricing recommendations, significantly improving market share and operational efficiencies.
Introduction
Problem Statement
A multi-billion-dollar US conglomerate with a global presence acquired a new LED product manufacturing organization, aiming for aggressive expansion and growth. Initially, the products were priced at a super-premium level to achieve a high ROI and market share. However, due to a lack of market awareness, the growth was stunted, forcing the company to rely on bespoke projects and managed services. Over three years, competition intensified, especially from Chinese manufacturers offering similar products at lower prices, leading to significant price erosion, reduced volumes, and a shrinking customer base.
Objectives
The primary objective was to develop a new market strategy and a dynamic pricing tool using the Tversky algorithm. This tool needed to provide rapid, data-driven pricing decisions, identify market gaps and opportunities, and streamline approval processes to enhance competitive positioning and operational efficiency.
Methodology
Full Assessment of Existing Pricing Process
An initial step involved a thorough assessment of the existing pricing process. The company’s pricing strategy relied heavily on manual processes using Excel spreadsheets, which were not only slow but also prone to errors. The approval process was cumbersome, involving multiple stakeholders and lengthy deliberation periods, often leading to delays that resulted in lost sales opportunities. This inefficiency was particularly detrimental given the high volume of requisitions—around 4,000 per day in one region alone.
Market Assessment
A comprehensive market assessment was conducted to understand the competitive landscape. This involved analysing competitors’ products, pricing strategies, service availability, market share, revenue share, growth targets, and aggressive strategies. The assessment also examined price discounts, rebate mechanisms, packaging options, and proliferation methods. Understanding these elements was crucial to identifying gaps and opportunities in the market.
Tversky Algorithm in Pricing
Background and Theory
The Tversky algorithm, named after cognitive psychologist Amos Tversky, is a model used for decision-making and similarity measurement. It is particularly effective in handling complex, multi-attribute decisions, making it ideal for dynamic pricing models. The algorithm’s foundation lies in behavioural economics, specifically Prospect Theory, which Tversky developed with Daniel Kahneman. This theory examines how people choose between probabilistic alternatives that involve risk, where the probabilities of outcomes are known.
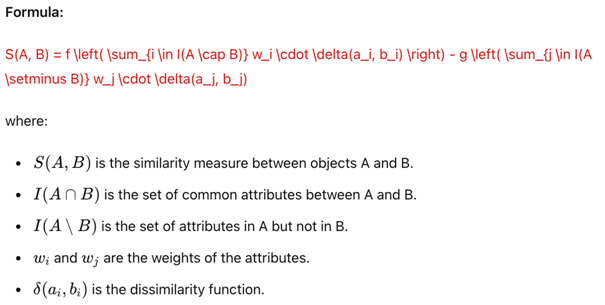
Application in Pricing Models
The Tversky algorithm was applied to develop a pricing tool that could analyse large volumes of data in real-time and provide optimal pricing recommendations. The tool considered various factors, including market conditions, competitor prices, historical sales data, and customer preferences. This enabled the sales team to make rapid, informed pricing decisions, significantly reducing the decision-making time and improving responsiveness to market changes.
SWOT Analysis
The SWOT analysis identified the company’s strengths, weaknesses, opportunities, and threats. Key strengths included advanced R&D capabilities, strong brand recognition, and a global presence. Weaknesses involved the initial lack of market awareness, slow manual pricing processes, and high product pricing. Opportunities were found in emerging markets, technological advancements in AI and ML, and the potential for bundled offerings. Threats included intense competition from low-cost manufacturers, rapid price erosion, and changing customer preferences.
Table: SWOT Analysis
Strengths | Weaknesses |
Advanced R&D capabilities | Initial lack of market awareness |
Strong brand recognition | Slow manual pricing processes |
Global presence | High product pricing |
Opportunities | Threats |
Emerging markets | Intense competition from low-cost manufacturers |
Technological advancements | Rapid price erosion |
Potential for bundled offerings | Changing customer preferences |
PESTEL Analysis
The PESTEL analysis provided insights into the external factors impacting the company’s strategy. Political factors included trade policies and regulations on energy efficiency. Economic factors involved global economic conditions and exchange rate fluctuations. Social factors considered increasing consumer awareness of energy-efficient products. Technological factors focused on advancements in LED technology and AI applications. Environmental factors examined regulations and sustainability trends. Legal factors included compliance with international trade laws and intellectual property protection.
Solution Development
Overview of Considered Solutions
Several solutions were considered, including traditional regression models, heuristic approaches, and advanced AI and machine learning algorithms. Each option was evaluated for its ability to handle the complexity and volume of pricing decisions required.
Justification for Tversky Algorithm
The Tversky algorithm was chosen due to its robustness in handling multi-attribute decision-making and its ability to model consumer behavior accurately. Unlike traditional regression models, the Tversky algorithm could dynamically adjust to market changes and provide real-time pricing recommendations.
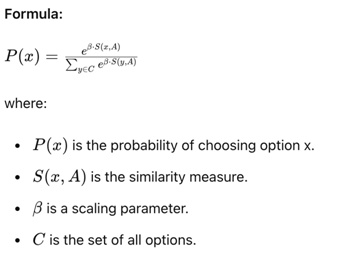
Strategy Implementation
Price Strategy
The new pricing strategy focused on competitive positioning and value-based pricing. This included dynamic adjustments based on real-time market conditions, strategic discounts, and customized pricing for different market segments. The tool’s AI capabilities ensured that pricing decisions were data-driven and aligned with market trends.
Promotions Strategy
Promotional activities were designed to enhance market penetration and customer engagement. These included targeted promotions, seasonal offers, and loyalty programs. By analysing customer data, the tool could suggest personalized promotions, increasing their effectiveness.
Channel Strategy
A comprehensive channel strategy was developed to optimize distribution and reach. This included identifying key distribution partners, optimizing supply chain logistics, and implementing channel-specific promotions and rebates.
Tool Development and Features
Architecture and Design
The pricing tool was designed with a modular architecture, integrating seamlessly with existing systems. The front-end provided an intuitive interface for sales teams, while the back-end leveraged advanced AI and ML algorithms for data processing and analysis.
AI and ML Integration
The tool integrated AI and ML to enhance its decision-making capabilities. Machine learning algorithms were used to analyse historical sales data and predict market trends, while AI algorithms provided real-time pricing recommendations based on current market conditions.
Business Case Creation
Use Case Scenarios
The tool supported various use case scenarios, including best-case, worst-case, and most-likely scenarios. It provided insights into competitor price positioning, market share analysis, and profitability calculations.
Impact Analysis
Impact analysis capabilities enabled the company to assess the potential impact of pricing decisions on market share and revenue. This allowed for informed decision-making based on comprehensive data analysis.
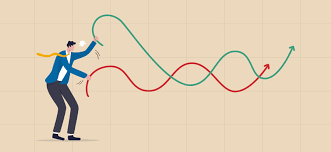
Results and Outcomes
The implementation of the Tversky algorithm-based pricing tool led to significant improvements in market share, revenue, and operational efficiency. Market share in the UK increased from 7.1% to 11.3%, and revenue growth exceeded initial targets. The tool streamlined the pricing approval process, reducing decision-making time from weeks to seconds and increasing order processing capacity from 4,000 to 11,500 orders per day.
Long-Term Strategies
Customer Retention
Long-term customer retention strategies focused on providing exceptional service, implementing loyalty programs, and engaging customers through personalized offers. The tool’s AI capabilities enabled continuous analysis of customer data to optimize retention efforts.
Value Generation
Value generation was prioritized by delivering high-quality products and comprehensive service packages. This approach ensured sustained customer satisfaction and loyalty.
Brand Positioning
Efforts to enhance brand positioning included consistent messaging, participation in industry events, and leveraging positive customer experiences. These strategies aimed to build strong brand recognition and loyalty.
Conclusion
The application of the Tversky algorithm in the pricing strategy of the conglomerate’s LED product line resulted in significant market share gains, operational efficiencies, and improved customer satisfaction. By leveraging advanced AI and machine learning technologies, the company was able to make informed, data-driven pricing decisions, enhancing competitive positioning and ensuring sustained growth. The success in the UK market provides a strong foundation for replicating the strategy in other regions, ensuring long-term success and market leadership.
The Tversky algorithm is a decision tree-based algorithm that uses the Tversky index to measure the similarity between two sets. It is known for its ability to handle asymmetric similarity judgments and has been applied in various domains such as image retrieval and recommendation systems.
In terms of accuracy, the Tversky algorithm has been shown to outperform other algorithms in certain applications. For example, in a study on image retrieval, the Tversky algorithm was found to be more accurate than other algorithms such as the Euclidean distance and the Cosine distance. Similarly, in a study on recommendation systems, the Tversky algorithm was found to be more accurate than other algorithms such as the Jaccard similarity and the Dice similarity.
In terms of speed, the Tversky algorithm is generally considered to be faster than other algorithms that use more complex models such as neural networks or support vector machines. This is because the Tversky algorithm is based on a simple decision tree structure that can be easily computed using a gradient descent optimization algorithm.
However, it is important to note that the accuracy and speed of the Tversky algorithm can vary depending on the specific application and the quality of the training data. Additionally, the Tversky algorithm may not be suitable for all applications, particularly those that require more complex models or more nuanced similarity judgments.
In the context of pricing algorithms, the Tversky algorithm can be used to determine the similarity between different products or services based on their features and attributes. This can be useful in applications such as product recommendation systems or price comparison websites. However, the Tversky algorithm may not be the best choice for all pricing applications, particularly those that require more complex models or more nuanced similarity judgments.
In summary, the Tversky algorithm is a decision tree-based algorithm that uses the Tversky index to measure the similarity between two sets. It is known for its ability to handle asymmetric similarity judgments and has been applied in various domains such as image retrieval and recommendation systems. In terms of accuracy, the Tversky algorithm has been shown to outperform other algorithms in certain applications. In terms of speed, the Tversky algorithm is generally considered to be faster than other algorithms that use more complex models. However, the accuracy and speed of the Tversky algorithm can vary depending on the specific application and the quality of the training data.